Some of us developed our musical palette around a milk crate full of vinyl. Others, a stack of cassette tapes or a stuffed binder of CDs. For years, receiving physical copies of classics from keen-eared relatives and friends was how we gained exposure to new influences. As we came into our own, maybe we heard something on the radio that grabbed our attention. Then we dropped a little money for the whole album, hoping that it held up beyond that hot new single. In 2020, the game has changed completely.
These days, we pay for participation, not property, and we’re swimming in recommendations. We have unprecedented access to a global catalog that we could never hope to exhaust. The internet is an always-open portal to unknown territories. And there are a ton of us taking advantage of this opportunity.
Breaking Down the Data
According to a report from MIDiA, a specialist media and technology analysis company, “streaming as a whole has grown in 2020 with 400 million subscribers.” Breaking that down, the report shows Spotify as the most popular platform worldwide, holding 32% of the total market share. Apple Music falls below that at 18%. Amazon Music claims 14%.
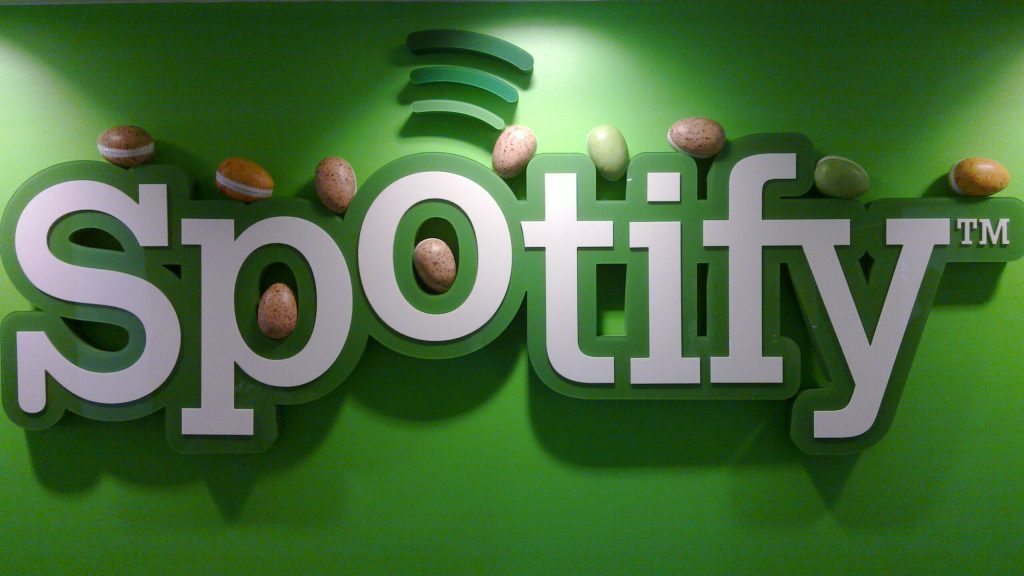
Three Platforms to Rule Them All
Other platforms are in play, of course. The same report shows that Tencent Music Entertainment holds 11% of the market. This figure is based largely on its popularity in China. Google Play Music and YouTube Music are growing, especially among younger audiences. Tidal still caters to its loyal audiophiles. Deezer and Pandora have their place. But the top three—Spotify, Apple Music, and Amazon Music—carry most of the weight.
With these popular platforms driving so much of what we listen to, it’s important to understand how they decide what we hear. To what extent are the algorithms in charge, and when do humans have a say? As you might expect, it varies by platform.
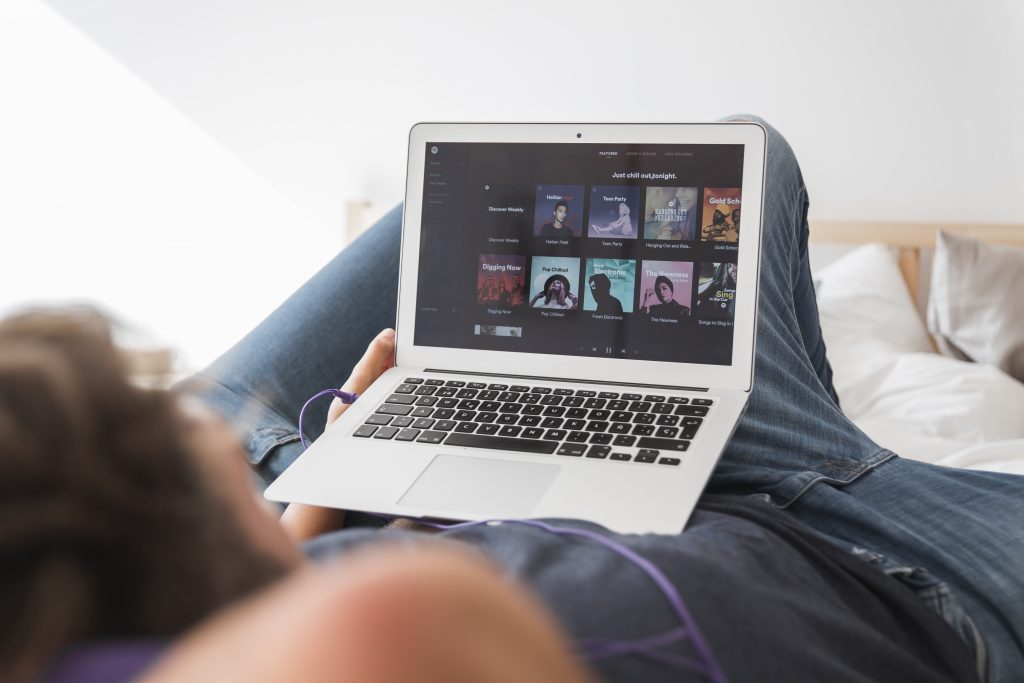
How Spotify’s Recommendations Work
Despite how much talk there is around the algorithms that drive Spotify’s recommendations, humans still have a hand in its magic. It all depends on what kind of playlist you’re listening to: algorithmic, editorial, or listener-created.
Algorithmic Playlists
Weekly Discoveries
The most widely discussed algorithmic playlist is “Discover Weekly”—a personalized, 30-song roundup of promising tracks delivered every Monday. Your listening history and preferences that Spotify has picked up on based on your past engagement, form the basis of this playlist. This is as you’d expect, but there’s more to it than meets the ear. “Discover Weekly” is a recommendation engine that applies three layers of logic: collaborative filtering, natural language processing, and audio analysis.
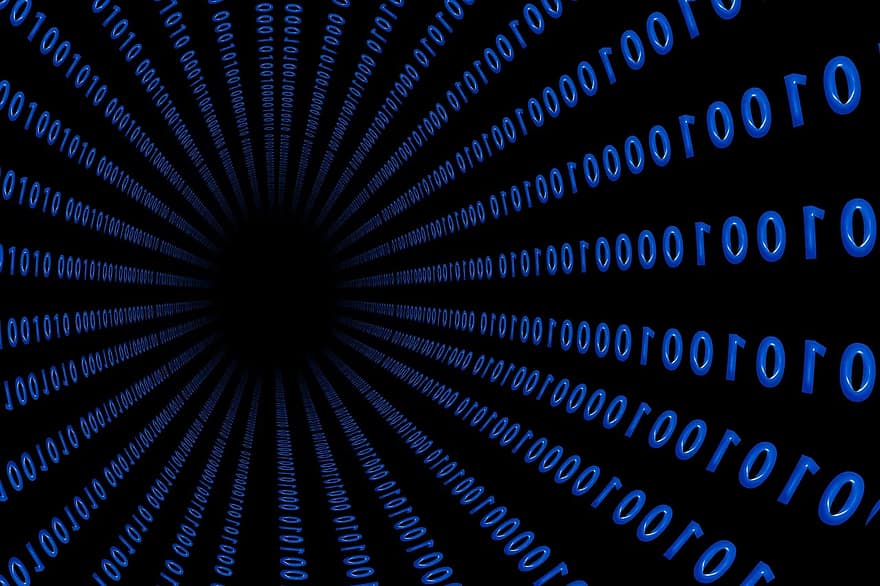
Collaborative filtering focuses on the similarities between your profile and profiles that overlap. If someone out there has similar taste and loves a song that you haven’t heard yet, chances are high that “Discover Weekly” will recommend that song to you.
“Discover Weekly” also applies natural language processing to articles, blogs, and metadata that refer to specific songs and artists. If an industry magazine profiles an artist or reviews a song, Spotify’s algorithm analyzes the text of that profile or review and updates its list of terms associated with that artist or song. The list changes daily and serves as a reference point for the Discover Weekly algorithm as it determines which songs are similar.
Finally, Spotify analyzes the actual audio of each file for characteristics that help it classify the song. These include tempo, time signature, loudness, and key. This last layer of analysis helps Spotify highlight songs that aren’t as popular or don’t have as much press, but that fit the profile of what you like.
They aren’t personalized for you,
but there’s a person behind them
with people like you in mind.
On Your Radar
The other big algorithmic playlist, “Release Radar,” is more straightforward. It highlights new releases that Spotify thinks you’ll like based on your activity. If you have a certain artist in regular rotation, you’re probably going to see that artist’s latest release in your list.
But the algorithms don’t make every decision.
Editorial Playlists
Spotify also offers a wide catalog of editorial playlists curated by experts. Some of these are specific to certain genres (such as “Rap Caviar” and “Rock This”) while others draw on moments and moods (such as “Power Workout” and “Focus Flow”). They aren’t personalized for you, but there’s a person behind them with people like you in mind.
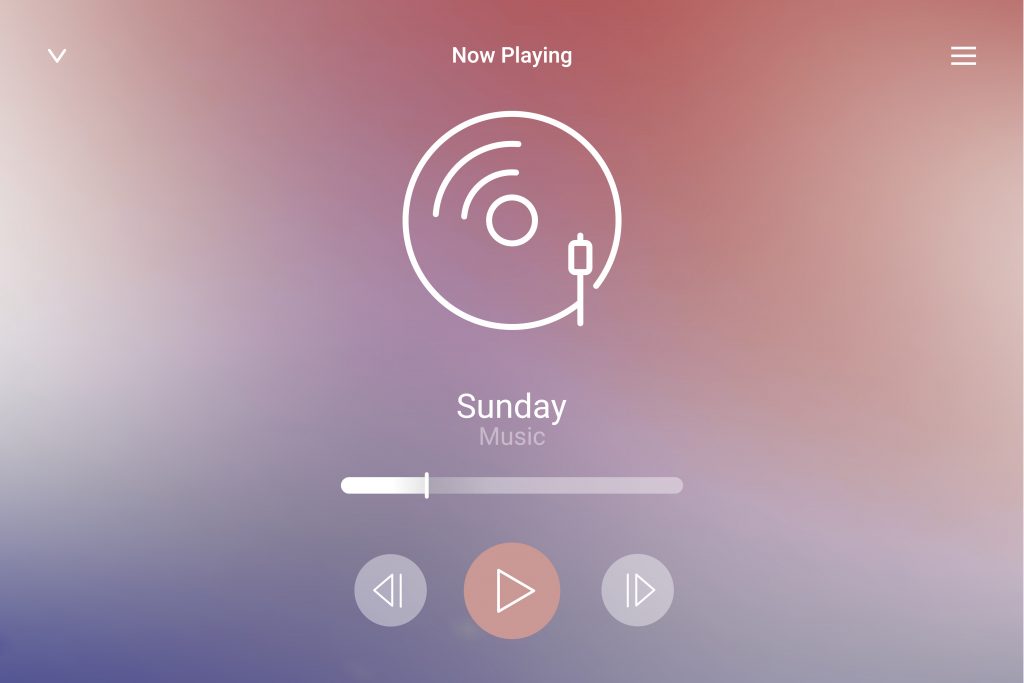
Listener-Created Playlists
The last playlist type, the listener-created playlist, is the new mixtape. These are the playlists that you and other Spotify listeners assemble. They are the public hours-long party playlist your savvy coworker put together. Perhaps it’s a private guilty-pleasures playlist you put on when you’re alone. It could be the anniversary playlist your partner surprises you with on the big day. Moreover, while branded playlists grow in popularity, most of these playlists come from everyday listeners.
How Apple Music’s Recommendations Work
Unlike Spotify, which was algorithm-driven right from the start, Apple Music as it exists today is an evolution of iTunes, and its automated recommendations have arrived and evolved over time.
An Expert Approach
In its early days, humans formed the basis of Apple Music. The launch announcement in 2015 alluded to the fact that you could “let Apple Music do the work for you” when you wanted to explore its 30 million songs. It didn’t mention any algorithms or automation.
Instead, the announcement noted that “Curation is the soul of every playlist created on Apple Music. Apple has hired the most talented music experts from around the world, dedicated to creating the perfect playlists based on your preferences, and they become better curators the more you listen.”
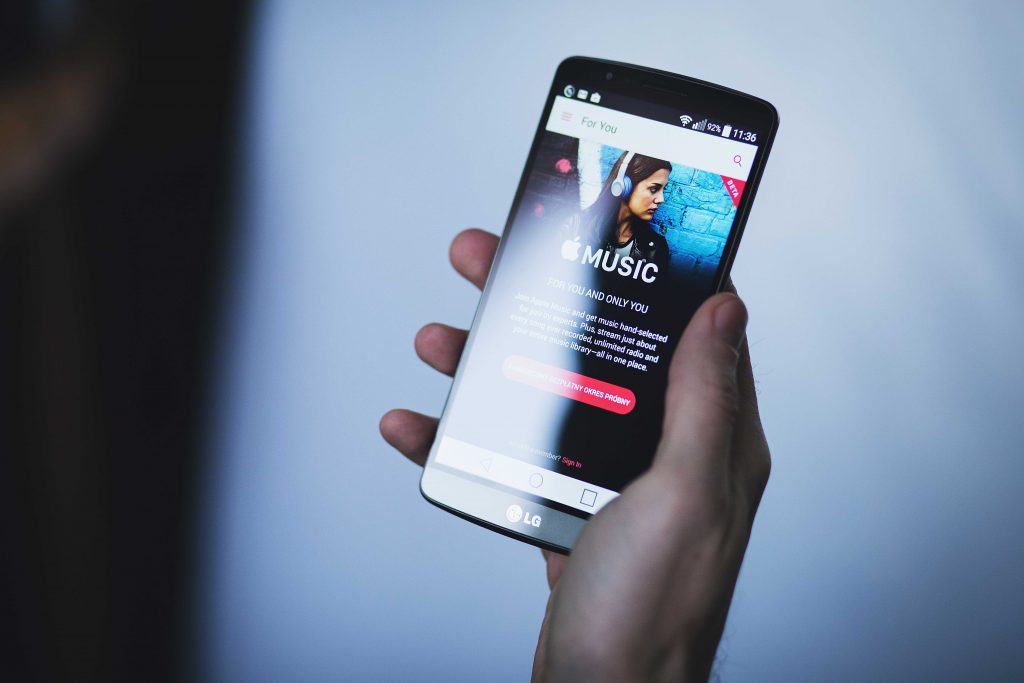
Real Genius
However, the Genius feature of iTunes had been around since 2008, when it launched with iTunes 8. That announcement referred to Genius as a breakthrough feature. It noted that the tool “lets you automatically create playlists from songs in your music library that go great together—with just one click. Genius helps music fans rediscover favorite songs in their existing music library and suggests related music on the iTunes Store they might want to add to their music collection.”
Genius at Work
How did Genius work? According to the announcement, “[I]nformation about your music library is anonymously sent to the iTunes Store. There, it’s combined with the anonymously-gathered knowledge from millions of other iTunes users and processed through Apple-developed algorithms. The Genius results, specifically tailored to your personal music library, return to your computer and enable users to automatically create Genius playlists in iTunes and on your iPods, even when they are not connected to the Internet.”
Lurking in the Shadows
So, while Apple Music was largely curated by humans, Genius was lurking behind the scenes. Then, with the release of iOS 10, Apple Music was completely redesigned. With the update came the addition of two algorithmic playlists: “My New Music Mix” and “My Favorites Mix.
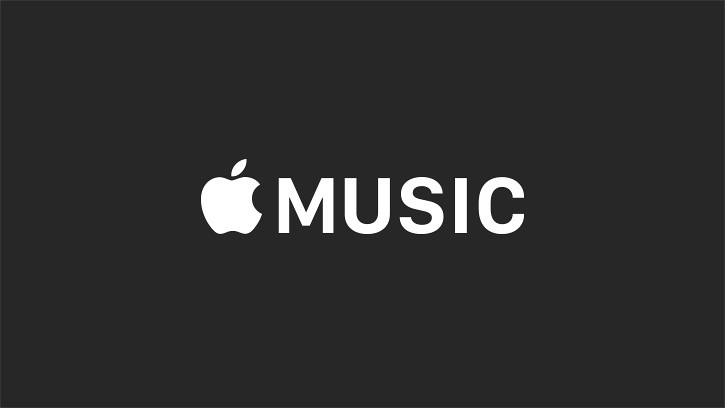
History in the Making
Your iTunes history creates the algorithms behind these new playlists. Songs and albums that had higher star ratings in your library—or that you played often—were factored into the recommendations in “My Favorites Mix.” If Apple Music identified that seemed to fit your profile but you hadn’t listened to it yet, it landed in “My New Music Mix.”
Apple plans to roll out more algorithmic playlists, but they’re taking a considered approach and keeping humans in the loop. In March 2020, Apple Music introduced the “Get Up! Mix,” which serves up a personalized mix of upbeat music that’s based on algorithmic recommendations and refined by human editors
How Amazon Music’s Recommendations Work
Of the top three streaming platforms, Amazon Music seems to be the least algorithm-driven. “My Discovery Mix” appears to be the most widely recognized playlist that offers automated recommendations.
You Get What You Paid For
However, the inner workings of “My Discover Mix” remain mysterious. That said, its recommendations appear pretty basic. For example, if you’ve bought music with your Amazon account, your mix will factor in those purchases and surface similar artists.
That does present some challenges. Based on my exploration, “My Discover Mix” doesn’t differentiate between music that bought for yourself and music that was bought as a gift. To that end, the majority of my recommendations came from a short list of CDs I bought my mom last year.
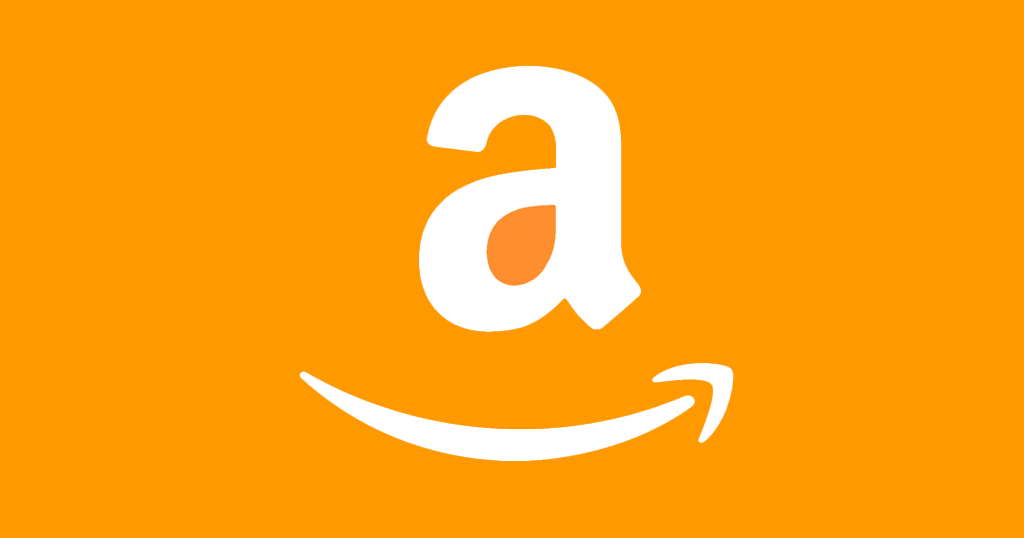
Curation Station
However, there’s an option to start at a song or album and explore “Customers Also Listened To,” which counts as algorithmic curation if you really stretch. Unfortunately, it doesn’t always yield results. When I tried to follow this path from one of the albums in my list, I got a full-screen error message. It read, “No Similar Recommendations,” and linked me back to the Home page. Other listeners have reported they are served a list of more songs by the same artist.
Given that Amazon has one of the most complex recommendation engines out there, it’s a bit of a letdown to get such limited results on Amazon Music.
“Alexa, Play Me a Song”
One area in which they’ve invested deeply is voice control. Naturally, the company that brought us Alexa designed its streaming service to take full advantage of the tool.
According to Amazon, you can “Find your music by asking Alexa to play a song using the title, artist, lyric, playlist, or station. You can also stay up to date on the news—just ask Alexa to play the Weekly One. In addition, if you just want something that fits your mood, Alexa’s got you covered. Just ask, ‘Alexa, play pop songs for cooking’ while you flip those pancakes or sauté that stir-fry.”
To some extent, requesting a mood-based playlist through Alexa involves algorithmic intervention. If you ask Alexa to play sad songs, for example, algorithmic metadata informs which playlist will play. (In my testing, I got a playlist called “I’m sad” that kicked off with Adele.) However, the playlists themselves are still mostly curated by the Amazon Music editorial team.
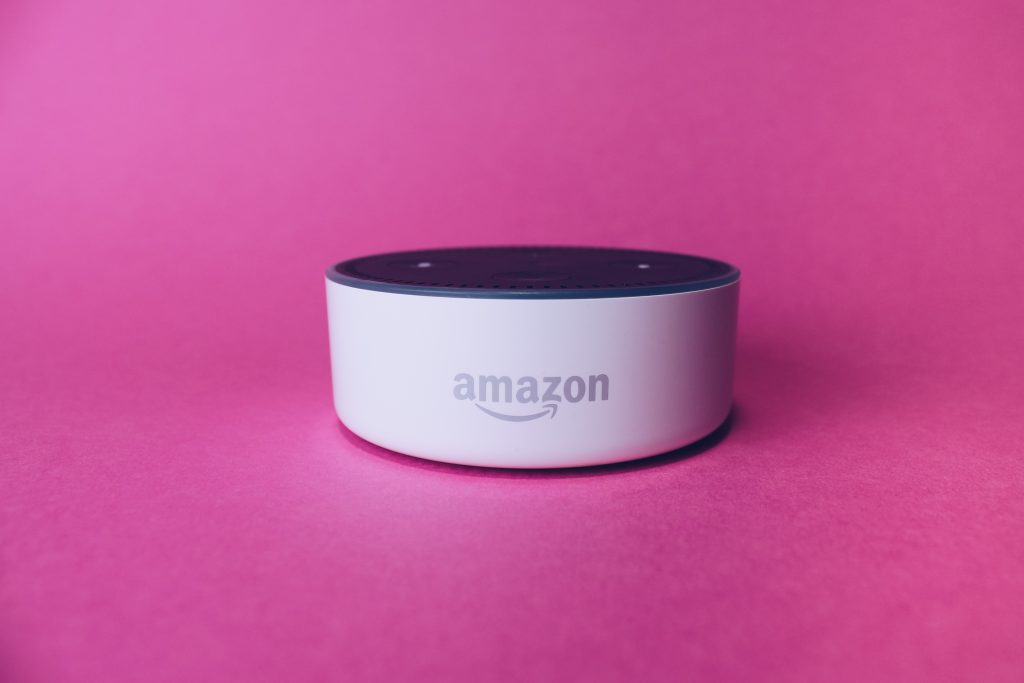
How do Algorithms Affect the Way We Listen?
Despite how much human influence we still find on the top three streaming platforms, algorithms still have a huge impact on what we hear and how we listen. Whether that’s good or bad depends on your perspective.
Breaking it Down
An Audioxide article from early 2019 took a close look at the way these streaming platforms—especially Spotify—are changing our habits. Let’s look at some of the article’s findings.
User Takeaways
- Discover new music on streaming platforms
- Listen to different genres
- Pay more attention to lyrics
- Make our own playlist
- Pirate less music
Musician Takeaways
- Independent artists more likely to grow
- Musicians more likely to feel the pressure
Pros and Cons
The Exploration Game
For those of us who love to explore new artists and genres (or who need a nudge to try something new), streaming helps us hear more of the world. Likewise, when we find something that we like, we’re more likely to tuck it away for later by saving it or creating a playlist. We’re also more likely to hear lesser-known artists who might have been beyond our reach in a world that’s limited by physical album sales and live shows.
That can help us reignite our curiosity and expand our palettes.
Keeping Up
On the flip side, discovery doesn’t always lead to profitability for the artists who create the music that we enjoy. Artists often find themselves adjusting their compositions and album structures to cater to shorter attention spans and playlist-driven listening habits.
Furthermore, algorithms rely on metadata to guide them, but the structure of that metadata favors some genres over others
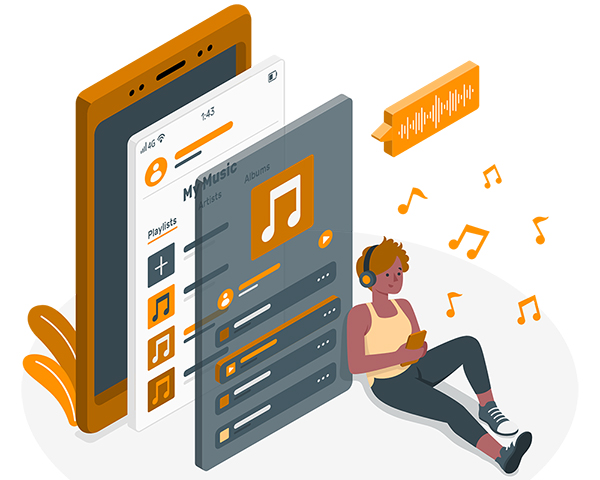
The Classical Conundrum
For example, exploring classical music doesn’t see as much success on streaming platforms. That may be because it doesn’t break down into standard metadata as easily. A New York Times article notes that, according to tracking service Alpha Data, “While 2.5 percent of album sales in the United States are classical music, it accounts for less than 1 percent of total streams.”
Why is classical music so difficult to quantify in a platform’s database? First, there usually more than one potential “artist” (composer, symphony, conductor, soloist, etc.). Next, movements don’t always translate cleanly to song titles. Finally, for people trying to listen to a specific movement by requesting through a voice assistant, figuring out what to ask for can become frustrating. This drives many listeners to give up.
Play to Pay
However, discovery can lead to higher ticket sales for touring artists, and data structures will improve over time.
Ultimately, it’s up to us to decide how much of our musical destiny we place in the hands of these algorithms.
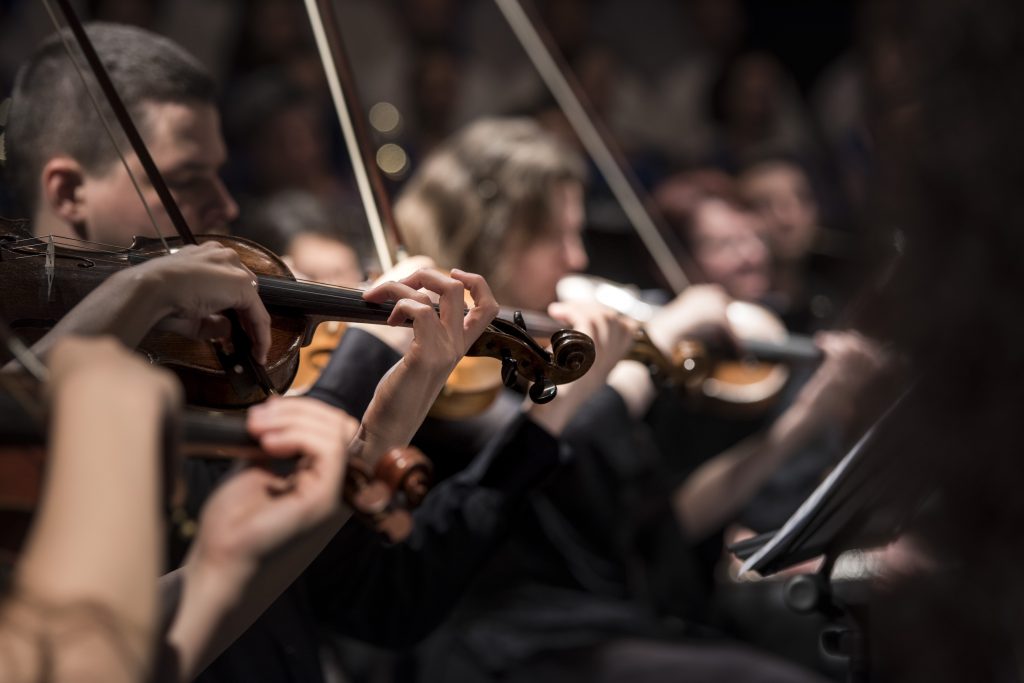